Understanding Screenshot OCR: Techniques and Applications
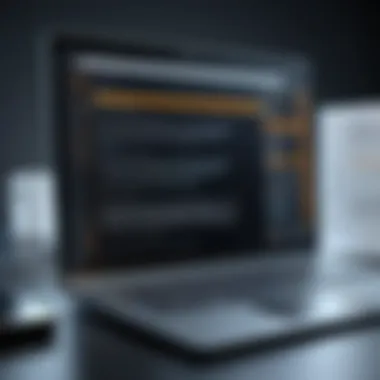
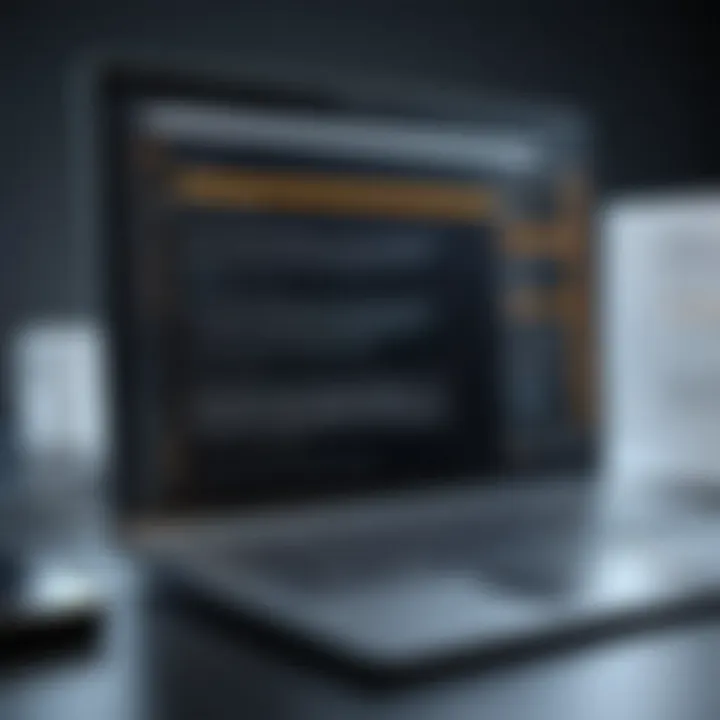
Intro
Optical Character Recognition (OCR) has evolved as a crucial technology in various sectors. Screenshot OCR, a subset of traditional OCR, allows users to extract readable text from images, screenshots, or photos. This capability is instrumental in enhancing productivity, bridging the gap between static images and dynamic text-based applications.
In this article, we will discuss the fundamental techniques of Screenshot OCR, explore its practical applications, and analyze its benefits and limitations. By the end of this exploration, readers will gain a comprehensive understanding of how Screenshot OCR integrates seamlessly into modern workflows and its impact on efficiency.
Key Features
Techniques of Screenshot OCR
Screenshot OCR employs several techniques that contribute to its functionality. At the core, the technology involves image preprocessing, text detection, and character recognition. Each step is vital to ensure accuracy and efficiency in text extraction.
- Image Preprocessing
The first step involves preparing the image for OCR. This might include adjusting brightness, contrast, and removing noise. A clear image significantly enhances the recognition process. Tools often utilize edge detection and binarization to improve performance. - Text Detection
After preprocessing, the OCR software identifies regions of the image that potentially contain text. This step is crucial as it determines where the software should focus its recognition efforts. It often includes techniques such as connected component analysis. - Character Recognition
This stage involves recognizing the characters present in the detected text regions. Modern OCR solutions often use machine learning and neural networks to achieve high accuracy rates. They can learn from vast datasets, improving their recognition over time.
Applications of Screenshot OCR
Screenshot OCR finds its way into various practical applications across industries. Some of the notable use cases include:
- Document Digitization
Individuals and businesses can digitize printed materials for easier access and storage. Screenshot OCR can quickly capture important information from documents and make it editable. - Language Translation
Programs like Google Translate can convert text from images to editable formats, allowing users to translate text from signs, menus, or documents on the go. - Data Entry Automation
In workplaces where manual data entry is time-consuming, Screenshot OCR automates the input process, minimizing human error and saving time. - Accessibility Enhancements
Screenshot OCR aids individuals with visual impairments by converting images to readable text, thus fostering inclusivity.
"The advent of Screenshot OCR simplifies access to information. Only a few clicks can convert static content into dynamic data."
Benefits of Screenshot OCR
The benefits of embracing Screenshot OCR technology are clear:
- Increased Productivity
By converting text from images, users can save time on manual entry or searches. - Enhanced Workflow Integration
Many Screenshot OCR tools integrate with existing software, such as cloud storage systems, enhancing collaborative efforts. - Cost-Effective Solution
Compared to traditional data entry or transcription services, Screenshot OCR can serve as a more economical option for businesses.
Limitations of Screenshot OCR
Despite its advantages, Screenshot OCR is not without limitations:
- Accuracy Issues
Poor image quality or unusual fonts can lead to lower accuracy rates. The technology may struggle with handwriting or artistic fonts. - Language Limitations
Certain OCR systems may not support all languages or language combinations. - Special Character Recognition
The ability to recognize special symbols or scientific notations is still a challenge for many OCR applications.
Prelude to Screenshot OCR
The rise of digital documentation has necessitated advanced tools for managing text. Screenshot OCR, or Optical Character Recognition, has emerged as a prominent technology that allows users to extract text from images or screen captures efficiently. Understanding the workings of Screenshot OCR is crucial for anyone involved with digital content, whether for personal use or within a business context.
Definition and Functionality
Screenshot OCR refers to the process of converting images, such as screenshots, into editable text. The functionality of Screenshot OCR lies in its ability to recognize characters from an image file using sophisticated algorithms. This technology is particularly useful for digitizing printed material, creating editable files from physical documents, and enhancing workflow by reducing manual data entry. Users can take a snapshot of a document or webpage and instantly convert it into a format that can be copied, edited, or searched. This streamlines tasks that would otherwise be labor-intensive and time-consuming.
Historical Context of OCR Technology
The journey of OCR technology began in the early 20th century. Initially, it was used for tasks like reading postal addresses and typesetting. The development of computing technology in the latter half of the century allowed for significant improvements in OCR systems, moving them from basic pattern recognition to more complex algorithms that utilize neural networks and machine learning.
With advancements in camera technology and image processing software, Screenshot OCR evolved to meet the needs of users in various environments, from corporate offices to educational institutions. The present state of Screenshot OCR benefits greatly from historical advancements, integrating machine learning techniques that enhance its accuracy and speed. Today, products like Adobe Acrobat and ABBYY FineReader exemplify the culmination of decades of research and development in OCR technology.
"OCR technology has transformed the way we handle information, making it accessible and manageable across various mediums."
Understanding the evolution of OCR helps to appreciate its significance in contemporary workflows. As the pace of digital transformation accelerates, technologies like Screenshot OCR will undoubtedly play an increasingly vital role.
How Screenshot OCR Works
Understanding how Screenshot OCR works is crucial for leveraging its capabilities fully. This section examines the fundamental elements that drive Screenshot OCR, highlighting the processes involved in converting images containing text into editable and searchable data. The effectiveness of these techniques significantly enhances productivity and streamlines workflows for users from various backgrounds, including business professionals and students.
Image Processing Techniques
Image processing is the first step in the Screenshot OCR workflow. This process involves several methods designed to prepare an image for text recognition. The quality of the image is paramount; thus, techniques like deskewing, which aligns the text within the image, and denoising, which removes unnecessary artifacts, are often employed.
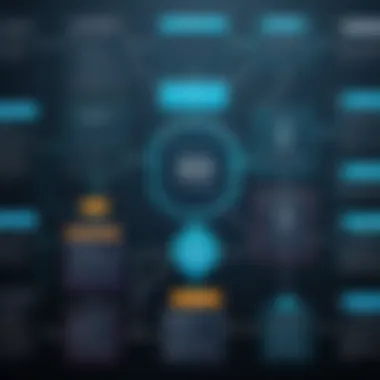
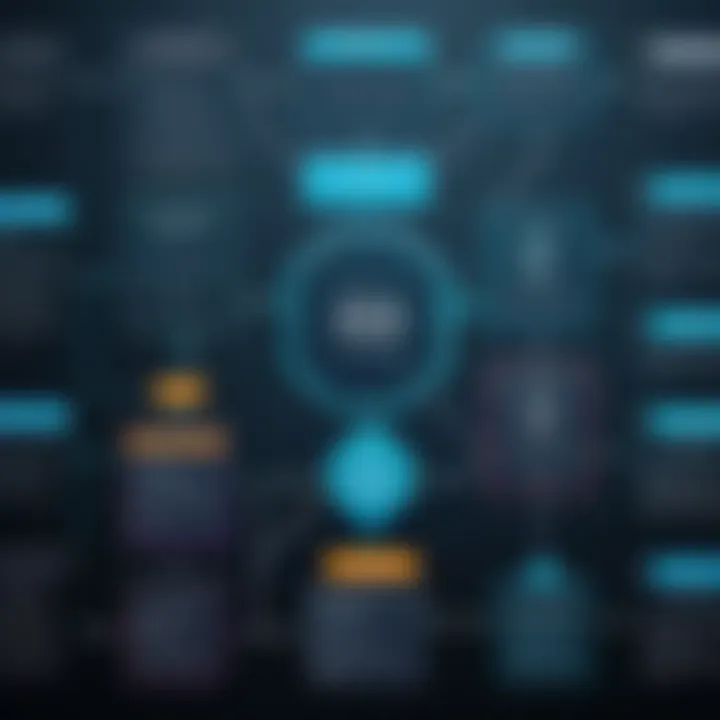
Additionally, binarization converts color images into black and white, improving the contrast between text and background. This is essential because OCR algorithms perform better with high-contrast images. The more processed an image is, the higher the likelihood of accurate character recognition. Efficient image preprocessing can lead to enhanced recognition results and reduce errors during text extraction.
Text Recognition Algorithms
The core functionality of Screenshot OCR hinges on sophisticated text recognition algorithms. These algorithms analyze the processed image to identify and convert text into machine-readable format. Two main types of text recognition algorithms are usually employed: traditional algorithms and neural network-based models.
Traditional algorithms, like Tesseract, utilize a series of techniques including pattern matching and feature extraction. On the other hand, neural networks, particularly convolutional neural networks (CNNs), provide higher accuracy by learning from vast datasets. CNNs partition input images into various segments to recognize patterns and shapes, allowing them to interpret complex fonts and varied handwriting styles. This adaptability makes neural network-based solutions particularly powerful in diverse applications.
"The choice of algorithm directly impacts the OCR performance in terms of efficiency and accuracy."
Machine Learning and AI Integration
The integration of machine learning and artificial intelligence into Screenshot OCR represents a significant advancement in the field. By employing these technologies, OCR systems can continually improve their performance over time. Machine learning algorithms enable the system to learn from previous inaccuracies, making them more adept at recognizing text under different conditions and contexts.
This innovation allows OCR technologies to adjust automatically to new fonts, languages, and formatting styles. Moreover, machine learning facilitates multi-language support, addressing a wide range of user needs globally. As organizations increasingly seek to digitize their processes, the application of machine learning techniques in OCR not only boosts operational efficiency but also enhances user experience by providing more reliable and accurate text recognition capabilities.
With these integrated approaches, Screenshot OCR technology continues to evolve, paving the way for more sophisticated applications and broader adoption in both personal and professional environments.
Applications of Screenshot OCR Technology
The significance of Screenshot OCR technology extends far beyond its basic functionality. This technology transforms text from images into a format that can be edited, searched, and disseminated. The applications of Screenshot OCR can enhance various sectors, from documentation to accessibility. Its adoption is driven by a need for efficiency and accuracy in managing information. As organizations and individuals increasingly rely on digital content, understanding these applications becomes pertinent.
Document Digitization
Screenshot OCR serves as a cornerstone for document digitization. Many businesses and institutions deal with vast amounts of paper documentation. By employing Screenshot OCR, these entities can convert printed files into digital formats. This process not only reduces the physical storage required but also simplifies information retrieval. The benefits include:
- Storage Efficiency: Transitioning to digital reduces the need for physical space.
- Searchability: Digital documents allow for instant searches, saving time.
- Preservation: Digitizing documents protects them from physical degradation.
In sectors like archive management and legal documentation, efficient digitization is crucial. Many organizations are now recognizing that digital archives are not just preferable; they are necessary for modern operations.
Data Extraction for Business Use
Businesses often face the challenge of extracting valuable insights from vast troves of data. Screenshot OCR facilitates data extraction by converting hard-to-edit documents, such as invoices or reports, into usable formats. This conversion supports several key functions:
- Improved Analytical Capabilities: By transforming unstructured data into structured data, businesses can analyze trends more effectively.
- Time Savings: Automating data entry frees employees from repetitive tasks, allowing a focus on higher-value activities.
- Increased Accuracy: OCR reduces the risk of manual entry errors, enhancing data quality.
With the rise of data-driven decision-making, enterprises are leveraging Screenshot OCR to yield valuable insights, streamline operations, and ensure data integrity.
Accessibility for Disabled Users
One of the fundamental applications of Screenshot OCR is in promoting accessibility for users with disabilities. The capability to convert images and documents into text formats can make information accessible to those reliant on assistive technologies. This technology allows:
- Screen Readers: Text generated by Screenshot OCR can be read aloud for visually impaired users.
- Customizable Formats: Documents can be formatted in ways that cater to individual needs, enhancing user experience.
- Enhanced Learning Opportunities: Educational institutions utilize Screenshot OCR to provide resources in accessible formats for students with disabilities.
Enhancing accessibility is not only a matter of compliance but also a step towards inclusivity. By integrating Screenshot OCR into their practices, organizations support diverse user needs, demonstrating a commitment to equal access to information.
"Accessibility means ensuring that everybody has equal access to information and technology. Screenshot OCR plays a vital role in this endeavor."
In summary, the applications of Screenshot OCR are manifold and impactful. From streamlining document management to fostering inclusivity, these technologies are reshaping how we interact with information in a digital age.
Popular Software Solutions for Screenshot OCR
Screenshot OCR technology has gained traction due to its utility in various sectors. This section delves into some of the most prominent software solutions available, touching upon their features, benefits, and practical considerations for users. Understanding these solutions can lead to better decision-making when selecting the right tool for specific needs.
Adobe Acrobat and its OCR Features
Adobe Acrobat is among the leading solutions for handling documents. Its OCR features are robust, enabling users to convert scanned documents into editable formats easily. By using Adobe’s technology, users can manipulate their documents' text in a way that maintains the original formatting. This approach is beneficial for legal documents, reports, and any other material where formatting is crucial.
- Key features include:

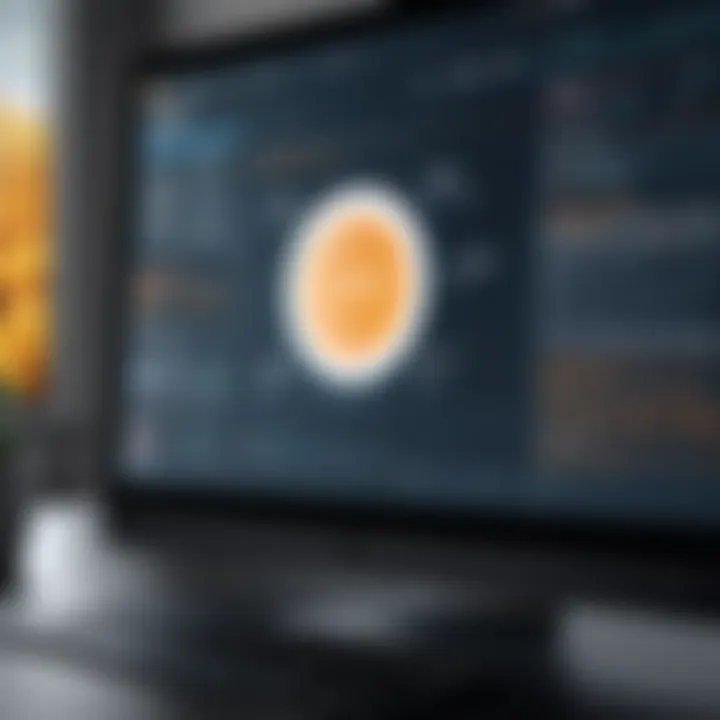
- Batch processing: Convert multiple files at once, saving time.
- Integration with other Adobe tools: Seamlessly connect with Adobe Sign and other applications to enhance workflow.
- Cloud storage options: Store documents securely while working across devices.
Overall, Adobe Acrobat offers a comprehensive set of tools for users who require advanced features in a user-friendly environment.
ABBYY FineReader Overview
ABBYY FineReader stands out for its sophisticated OCR capabilities. It is designed to provide high accuracy and speed. FineReader supports multiple languages and can handle various file formats, making it versatile. Users interested in digitizing paper documents or extracting information from screenshots find it particularly efficient.
- Noteworthy aspects of ABBYY FineReader include:
- Advanced layout retention: The software maintains the look and feel of the original documents.
- Document comparison feature: Easily identify changes between versions of a document.
- PDF editing capabilities: Modify PDF files directly without needing separate tools.
This tool caters to professionals who need precision and reliability in data handling.
Open Source Alternatives
For those seeking cost-effective solutions, there are several open-source OCR options available. These alternatives are not only free but also feature a community-driven development that can cater to specific needs.
Some popular open-source tools include:
- Tesseract: A powerful OCR engine backed by Google, suitable for developers who wish to integrate OCR functionality into their applications.
- OCRmyPDF: This tool provides an opportunity to add OCR text layers to scanned PDF documents, making them searchable and easier to navigate.
Considering open-source options can offer flexibility and customization. However, users may need to invest time in learning the software and troubleshooting issues without dedicated support.
"Choosing the right OCR software hinges on evaluating specific needs against the functionality offered by different solutions."
Advantages of Using Screenshot OCR
Screenshot OCR technology brings a variety of advantages that can significantly enhance productivity and efficiency across different fields. Its ability to convert images and screenshots into editable, searchable text has profound implications for businesses, educators, and everyday users. This section elaborates on the specific benefits of this technology, providing insight into why it has become increasingly essential in modern digital workflows.
Efficiency in Information Retrieval
The primary advantage of Screenshot OCR lies in its capacity for efficient information retrieval. Users frequently deal with vast amounts of data, and manually filtering through these is time-consuming. With Screenshot OCR, textual information embedded in images can be quickly extracted. This ability reduces the time needed to locate and reference information from documents that are only available in image format.
For example, professionals in legal fields often encounter documents in PDF or image formats. Instead of manually transcribing or searching through each document, they can employ Screenshot OCR tools to pull relevant text instantly. This drastically enhances the workflow, allowing users more time to focus on analysis and decision-making rather than data entry.
Reduction of Manual Typing Errors
Another significant advantage is the reduction of typing errors. Human error is inevitable in any manual input scenario. Screenshot OCR minimizes this risk by converting text directly from images into editable formats. This is particularly useful in data-sensitive fields like finance and healthcare, where errors can have substantial consequences.
Using Screenshot OCR ensures that the transcribed text mirrors the original accurately. It eliminates the discrepancies that often arise from typing, thereby maintaining the integrity of the information. Moreover, this capacity for accuracy can lead to higher levels of trust in the data processed, which is crucial for maintaining databases or records.
Enhanced Data Sharing Capabilities
Screenshot OCR also facilitates enhanced data sharing capabilities. In an increasingly collaborative environment, being able to extract and share information easily is invaluable. Users can transform screenshots or scanned documents into workable text files that can be edited, annotated, and shared across teams.
This versatility allows for not only real-time collaboration but also seamless integration with various applications. For instance, a designer might take a screenshot of a web page's text, utilize Screenshot OCR to convert it into an editable form, and then share it with the content team for immediate feedback. As a result, projects can progress more swiftly, enabling faster iterations and improved outcomes.
The adoption of Screenshot OCR can lead to better data handling and communication in teams, enhancing overall productivity.
In summary, the advantages of using Screenshot OCR are numerous. From speeding up information retrieval to reducing manual errors and enhancing collaboration capabilities, this technology serves as a crucial tool in today’s digital landscape.
Limitations of Screenshot OCR Technology
Understanding the limitations of Screenshot OCR technology is critical to grasping its full scope and potential. While OCR has advanced significantly in recent years, it is still not without challenges that users and developers must address. This section outlines specific concerns regarding accuracy, dependence on image quality, and issues related to language and character recognition.
Challenges with Accuracy
Accuracy remains one of the foremost challenges in Screenshot OCR technology. Despite advancements in machine learning and image processing, OCR systems often struggle with correctly interpreting text. Errors can arise in various forms, including character misrecognition, where letters may be confused with similar-looking ones. For instance, the letter "O" might be read as a zero or an "O" may be mistaken for an "Q". This inconsistency can lead to a range of issues, from minor inconveniences to significant errors in critical documents.
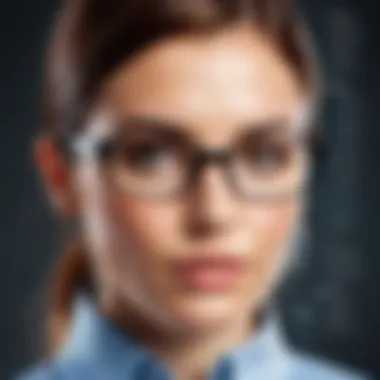
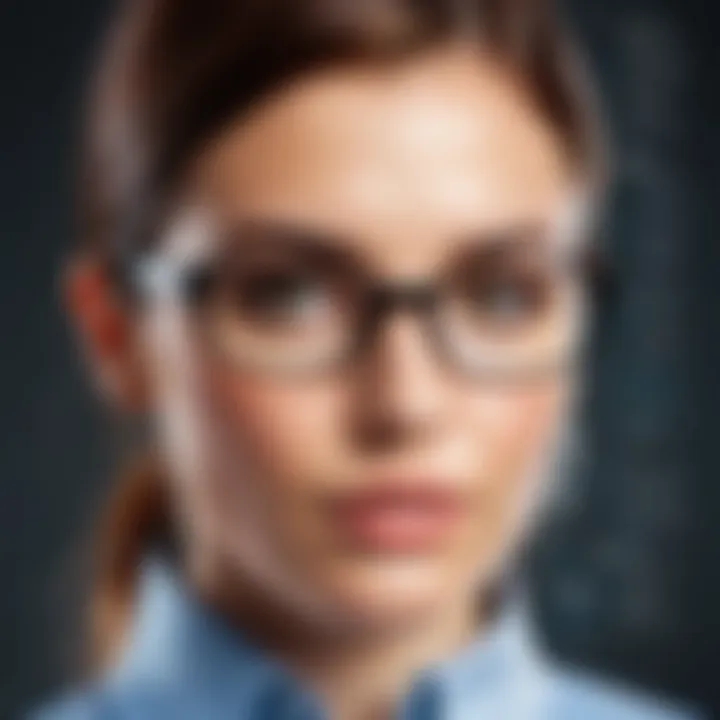
Users must also consider that the accuracy of OCR can fluctuate based on the complexity of the text. For example, documents with varied fonts, sizes, and styles pose additional challenges. Handwritten text, in particular, continues to be an area where OCR has not yet perfected its capabilities. Thus, understanding the potential for errors is essential when relying on Screenshot OCR for any serious applications, especially in legal or medical contexts where precision is paramount.
Dependence on Image Quality
Image quality is a crucial factor affecting the performance of Screenshot OCR technology. A clear and high-resolution image enhances the chances of accurate text recognition. Conversely, low-quality images—blurred, poorly lit, or low resolution—tend to yield substandard results. The reliance on image quality means that users must take care to provide the best possible screenshot or image source for optimal performance.
Beyond just resolution, the context of the image matters. Background noise, like cluttered images, or contrast issues between text and background can obstruct recognition attempts. Moreover, varying lighting conditions during image capture play a role, as shadows and reflections can distort text. Addressing these aspects is vital for anyone utilizing OCR in their workflow. Users must ensure that the quality of input images meets specific standards to prevent data loss or the need for extensive manual corrections.
Language and Character Recognition Issues
Language and character recognition issues present another significant limitation for Screenshot OCR technology. While many OCR systems support multiple languages, the accuracy of text recognition can vary widely among different languages. Some languages, especially those that do not use Latin scripts, can be particularly problematic. This can include languages such as Mandarin, Arabic, and various Cyrillic derivatives.
For complex scripts that feature contextual shapes for letters or diacritical marks, OCR performance may disappoint users. Moreover, slang, colloquialisms, or specialized terminology in niche languages often lead to misunderstandings or misinterpretations within the recognized text.
It's also important to note that not all Screenshot OCR tools are optimized for all languages equally. Users seeking robust multi-language support should conduct thorough research on available options. Ensuring compatibility with specific linguistic needs can be critical, especially for businesses that operate on a global scale and require accurate document processing in various languages.
In summary, while Screenshot OCR technology offers benefits, users must remain aware of its limitations. Addressing accuracy issues, maintaining high image quality, and being mindful of language capabilities are paramount for making effective use of OCR solutions.
Future Trends in Screenshot OCR Development
The landscape of Screenshot OCR technology is evolving rapidly. Understanding the future trends in this field is essential for tech-savvy individuals and businesses alike. As the capabilities of machine learning and artificial intelligence continue to advance, Screenshot OCR is becoming more accurate and versatile. This section will analyze some key developments and considerations that will shape the future of Screenshot OCR.
Advancements in Machine Learning Algorithms
Recent advancements in machine learning algorithms are central to improving Screenshot OCR technology. These algorithms analyze vast amounts of visual data to enhance text recognition capabilities. One notable direction is the shift towards deep learning techniques.
Deep neural networks are now able to learn patterns and features from images more effectively than traditional OCR methods. This reduces the rate of errors significantly, especially in complex font styles or layouts.
Moreover, pre-trained models such as Convolutional Neural Networks (CNNs) are being employed. They can be adapted to recognize new languages and character sets with ease. As these algorithms improve, Screenshot OCR technology can provide more reliable results across diverse applications.
Integration with Cloud Technology
The integration of Screenshot OCR with cloud technology is another promising trend. Cloud computing enables the storage and processing of large datasets off-site. This means that users can access powerful OCR services without the need for high-performance local hardware.
Cloud-based Screenshot OCR solutions offer several advantages:
- Scalability: Businesses can expand their OCR capabilities as needed without significant investments in infrastructure.
- Collaboration: Teams can work together more efficiently, accessing shared documents with text extracted from images in real-time.
- Cost-effectiveness: Users are less burdened by expensive software and hardware setups, thanks to subscription-based models.
This shift to cloud solutions enhances not only performance but also user experience, making advanced OCR technology accessible to a wider audience.
Potential Use in Augmented and Virtual Reality
Finally, the potential use of Screenshot OCR in augmented and virtual reality (AR/VR) environments presents exciting possibilities. As AR and VR technologies grow more sophisticated, integrating Screenshot OCR can create interactive experiences. Users can capture text from their surroundings to interact seamlessly with it.
For instance, imagine a scenario where a user points their device at a book page in VR, and the text is instantly converted into a digital format. This could facilitate educational tools, translate foreign languages in real-time, or enhance navigation through contextual information.
In summary, as machine learning algorithms advance, cloud technology integrates, and OCR finds applications in AR and VR, the future of Screenshot OCR will likely be marked by increased efficiency and wider applicability. Keeping abreast of these developments is vital for those looking to leverage Screenshot OCR technology in personal or professional contexts.
Epilogue
In reviewing the significance of Screenshot OCR technology, it becomes clear that this tool plays a pivotal role in enhancing productivity across various sectors. The technology simplifies the extraction and conversion of text from images into editable formats, which proves invaluable for users handling documents in diverse settings.
Summary of Key Insights
The exploration of Screenshot OCR leads to several critical insights:
- Efficiency: The ability to quickly convert images to text reduces the time spent on manual entry. This efficiency can lead to substantial productivity gains.
- Versatile Applications: From document digitization to enabling accessibility for individuals with disabilities, the applications of Screenshot OCR are broad and impactful.
- Advancements: Continuous improvements in machine learning and AI integration are enhancing the accuracy and functionality of OCR technologies.
- Considerations: Despite its benefits, users must be aware of the limitations related to image quality and character recognition challenges.
Sharing insights like these with tech enthusiasts and business professionals is essential. They help to understand how leveraging Screenshot OCR can significantly streamline workflows.
Implications for Users and Businesses
The implications of Screenshot OCR technology for both individual users and businesses are profound.
- Enhanced Productivity: For users, the ability to convert screenshots or images to text makes tasks like data gathering and documentation processes far easier and faster.
- Cost-Effectiveness: For businesses, utilizing Screenshot OCR can lead to reduced labor costs. By minimizing manual data entry, companies can allocate human resources more effectively.
- Competitive Edge: Companies that embrace this technology can operate more efficiently than competitors who do not leverage OCR tools.
- Improved Accessibility: Screenshot OCR significantly aids in creating a more inclusive environment for individuals with disabilities, providing them the tools needed to engage with content more readily.
In essence, as OCR technology evolves, both users and businesses must keep informed about its advancements. Adapting to such technologies can yield improvements in efficiency and operational capabilities.