Deep Reverse Image Search: Mechanisms and Uses
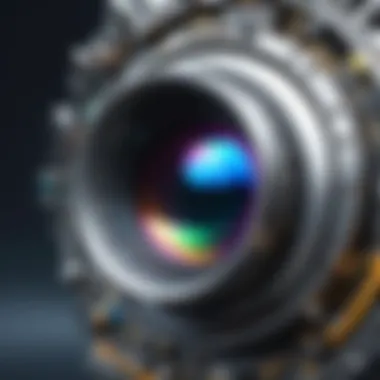
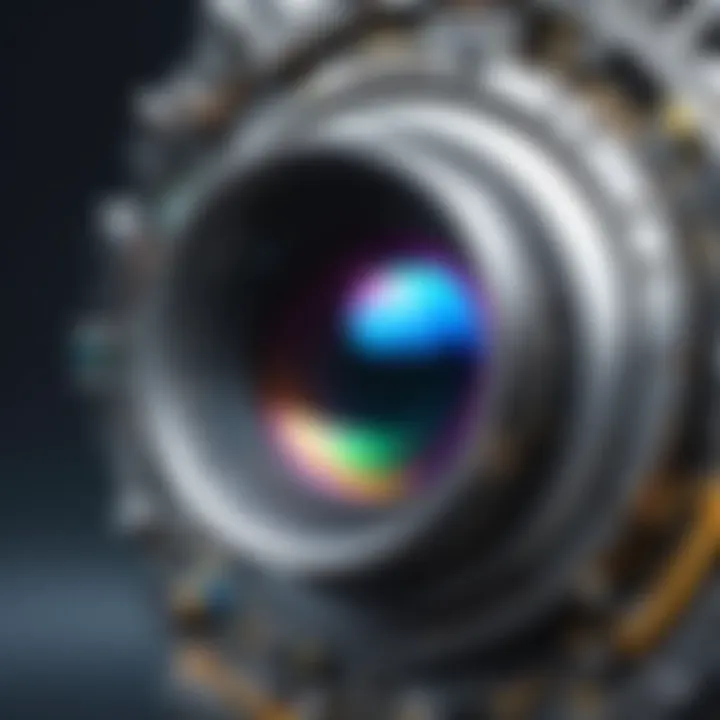
Intro
In an increasingly visual world, the significance of image-based search capabilities has never been more pronounced. Traditional search methods, which heavily relied on textual descriptions, often fall short when it comes to understanding the true essence of an image. Enter deep reverse image search, a groundbreaking tool that leverages the power of artificial intelligence and machine learning. This technology digs deeper than the surface, analyzing the content of images to deliver results that are not only relevant but also nuanced and contextually aware.
The power of deep reverse image search lies in its sophistication, harnessing algorithms that assess attributes like color, texture, and shapes within images. Unlike a basic search where keywords play a pivotal role, this advanced method transforms how we interact with visual content.
Understanding the mechanisms and applications of deep reverse image search can benefit a plethora of sectors, ranging from marketing to social media, enhancing how individuals and businesses utilize visual data. However, as with any innovative technology, it comes with its own set of ethical considerations and limitations, urging users to tread carefully.
This exploration will unpack the core features of this technology, providing insights into its design architecture and practical applications, while examining the critical considerations surrounding its use.
Understanding Reverse Image Search
In the digital era, a picture is more than just a fleeting moment captured; it has morphed into an efficient repository of data. Understanding the intricacies of reverse image search is key to leveraging this potent tool effectively. This technology allows users to conduct searches through images rather than keywords, fundamentally changing how we access and digest visual information. It opens doors to numerous possibilities, from identifying artwork to tracking down the source of a viral meme.
Definition and Basic Functionality
Reverse image search is a process where an image is used as the primary input for an inquiry, rather than relying on text-based keywords. Essentially, the user uploads an image, and the search engine processes that image to retrieve visually similar images, information associated with the image, or contextual data leading to the image's origin. This functionality taps into complex algorithms and databases to streamline the search process.
To understand how this all works, one can break it down into a few core steps:
- Image Acquisition: The user selects an image from their device or the web.
- Image Processing: Advanced algorithms analyze the image for distinct features. This can include colors, shapes, textures, and patterns.
- Database Querying: The processed image is then compared against countless images in existing databases.
- Result Generation: Finally, the system returns a list of visually similar images or related content.
Through this intricate dance of technology, reverse image search identifies and retrieves data that might not be apparent through text alone.
Evolution of Image Search Technologies
The roots of image searching can be traced back to early computers, but the methods of searching for images have come a long way. Initially, image searches relied heavily on metadata and manual input. Those days are long gone, as contemporary search engines leverage remarkable advancements in AI and machine learning to enhance image retrieval.
Key milestones in this evolution include:
- Early Image Retrieval Systems: These employed basic color histogram comparisons or simple keyword tags, which resulted in limited accuracy.
- Development of Content-Based Image Retrieval (CBIR): This method began analyzing the content of the images rather than just relying on their metadata.
- Introduction of Machine Learning: The shift toward using AI brought about improvements in how features are extracted and compared. Image search systems can learn over time, refining their accuracy and broadening their capabilities.
Now, technologies such as convolutional neural networks (CNNs) have revolutionized how images are processed. This advancement has enhanced the ability to classify images, ensuring greater relevance in search results, which ultimately benefits both consumers and businesses.
"With reverse image search, what you see is no longer limited to just the image on your screen; it's an invitation to explore a vast digital ecosystem."
The journey of reverse image search exemplifies how technology continuously adapts to the needs of users, paving the way for more seamless interactions in our increasingly visual world.
The Mechanism Behind Deep Reverse Image Search
Understanding how deep reverse image search operates is crucial to grasp its significance in a world dominated by visuals. This section explores the nitty-gritty of the technologies that power this advanced search mechanism, making it indispensable for both consumers and businesses.
Artificial Intelligence and Machine Learning
In the realm of deep reverse image search, artificial intelligence (AI) and machine learning (ML) are the heavyweights at play. They enable systems to sift through a mind-boggling amount of images to deliver pinpoint accuracy in search results. Essentially, these technologies train the system to recognize patterns and features within images.
Using neural networks, particularly convolutional neural networks (CNNs), the software can learn from millions of images. For instance, a well-designed CNN might be trained on thousands of pictures of dogs. As a result, when a user uploads a photo of their own pooch, the system can swiftly analyze it and match it against its extensive database. Not just any matching process, but a deep semantic understanding of the image content is achieved.
The use of deep learning takes it a step further, allowing for a more nuanced interpretation of image characteristics. It’s not merely a game of finding similar pixels but understanding shapes, colors, and even the context of the objects in the image.
This leads to a more refined result, enhancing user experience significantly. As more data is processed, these systems only get better, creating a cycle of improved accuracy and relevance in image retrieval.
Feature Extraction Techniques
Another critical aspect of deep reverse image search is feature extraction. This defines how the system perceives and processes images to retrieve similar ones. By breaking down an image into various attributes—such as edges, textures, and colors—the search engine creates a feature vector for each image.
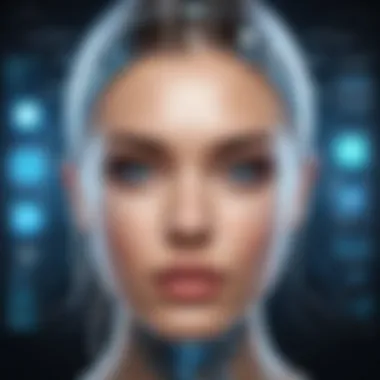

These vectors serve as distinct fingerprints for each image. When a user uploads an image for reverse search, the system computes its feature vector and performs distance calculations against the vectors in the database. There are several techniques for feature extraction:
- Histogram of Oriented Gradients (HOG): This visual descriptor is great for detecting objects within images.
- Scale-Invariant Feature Transform (SIFT): This extracts features invariant to scale and rotation changes, giving it a knack for identifying objects from different angles.
- Color Histograms: By representing colors in a histogram format, the system can recognize dominant colors in images, aiding in similarity measures.
These techniques form the backbone of how images are categorized and retrieved, thus playing an instrumental role in deep reverse image searches.
Similarity Measures in Image Retrieval
To ensure precise matching, similarity measures are employed following feature extraction. Once the system has the feature vectors for all images, it must determine which ones are more alike to the input image.
Several algorithms are utilized to calculate similarity, each with its strengths and weaknesses. The most common include:
- Euclidean Distance: A straightforward measure to calculate the root of the sum of squared differences, effectively determining how close two vectors are.
- Cosine Similarity: This gauges the cosine of the angle between two vectors, ideal for text or images with similar orientations.
- Jaccard Index: This metric compares the similarity of two sets, often used when dealing with binary images.
"The distance shared between the feature vectors dictates how closely related the images are, making similarity measures an essential puzzle piece in reverse image searching."
Using these measures, the system can generate a ranked list of similar images, allowing users to explore results that are contextually relevant. These underlying principles of deep reverse image searching illuminate its efficacy and adaptability in our increasingly visual-centric digital landscape.
Applications in Consumer Technology
Deep reverse image search is making waves in consumer technology, blending intelligence and usability. It's like having a smart assistant that knows a little more about what’s in your pictures. This capacity to analyze images can significantly improve how consumers interact with the digital world and even empower businesses to meet consumer needs more effectively.
E-commerce and Product Identification
Imagine you're out and about, a product catches your eye. You pull out your phone and take a snap of it. With deep reverse image search, you can instantly find where to buy that item, compare prices, or check out reviews—all at your fingertips. E-commerce platforms such as Etsy and Amazon benefit hugely from this capability. By utilizing sophisticated algorithms, these platforms can identify products from images that users provide.
- Shoppable images: Users can upload photos of items they like, leading them directly to purchase options.
- Price comparisons: This feature helps customers find the best deals by scanning a single image across various retailers.
- Boosting local businesses: Small shops can benefit by showing their goods online. A quick scan might reveal unique local treasures effectively.
Social Media and Content Verification
Social media is a landscape rich in imagery, but navigating it often requires knowing the source or understanding the context of an image. Deep reverse image search steps in here like a trusty compass. One can track the origin of a meme or the authenticity of a photo claiming to show a recent event.
- Rooting out misinformation: With the surge of viral images, ensuring their validity has become crucial. Algorithms dig through vast databases to find matches, helping users cross-check information before sharing.
- Promoting original content: Creators can track unauthorized use of their images across platforms, leading to better awareness of intellectual property.
Personal Photo Management
How many times have you scrolled through your photo library, trying to recall where a picture was taken or when? This is where deep reverse image search shines. It can help organize digital galleries and even revive forgotten memories.
- Automatic tagging: Advanced systems can automatically tag images based on their content—think vacations, family gatherings, or that random delicious meal that you snapped but forgot about.
- Memory recall: Picture a situational recall where you can enter keywords like "beach" or "birthday" into your photo management app. The system digs through your garage of images to find the ones you’re looking for.
- Simplified curation: With easy retrieval of images, crafting albums or slideshows becomes a breeze, saving time and effort.
In today's fast-paced world, technologies that ease daily tasks and enhance user experience are not just amenities but necessities.
Overall, the applications of deep reverse image search in consumer technology showcase its potential to transform how we shop, validate information, and manage personal memories. As consumers become increasingly tech-savvy, the demand for these features will only grow, pushing for further innovations.
Benefits of Deep Reverse Image Search
The world is overflowing with images, and the ability to accurately and efficiently search through them is crucial. Deep reverse image search offers a plethora of benefits that go beyond simple visual matching. In this section, we'll explore some key advantages that make this technology invaluable in various contexts, particularly for tech enthusiasts and professionals alike.
Enhanced Accuracy in Identification
When it comes to identifying objects or content within an image, the precision of deep reverse image search stands out. Traditional image searches often rely on mundane metadata or color matching, which can lead to misleading results. In contrast, deep reverse image search delves deeper, studying intricate features, patterns, and contextual cues in images.
To illustrate, think of a scenario where a consumer spots a pair of shoes in a street photo. Using conventional search techniques, finding that specific model might involve scrolling through pages of irrelevant content. With advanced algorithms, however, a user can upload the image directly and receive highly relevant results in moments. This capability not only saves users from frustration but significantly enhances their overall experience while searching.
Broader Image Database Access
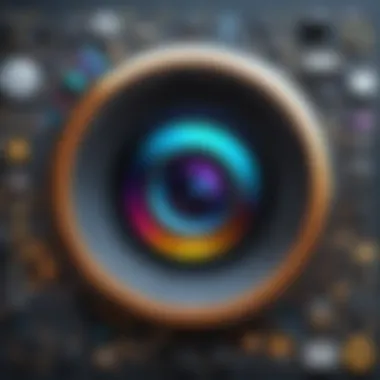
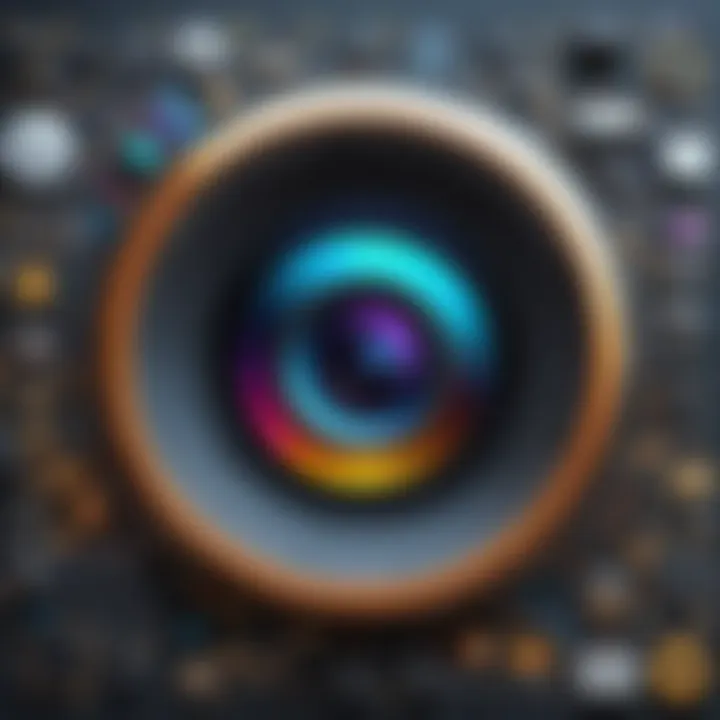
One of the crowning glories of deep reverse image search is its capacity to tap into expansive databases filled with diverse image content. Unlike basic search tools that often restrict searches to well-known platforms or repositories, advanced systems can scour a wider range of sites.
This feature proves exceptionally beneficial in various domains, such as:
- Research and Academia: Scholars can locate lesser-known images relevant to their studies.
- E-commerce: Shoppers can find products from various platforms, ensuring they don’t miss out on desirable options.
- Art and Culture: Enthusiasts can explore art pieces across museums and galleries globally, enriching their appreciation and knowledge.
By breaking down the walls that previously limited search capabilities, this technology empowers users to uncover a treasure trove of visual information, making their search endeavors much more fruitful.
Time Efficiency in Image Searches
In our fast-paced digital age, time is often a luxury we can't afford. Deep reverse image search transforms how quickly we can find information. Instead of dedicating countless hours sifting through irrelevant results or conducting repetitive searches, users can achieve astonishing speed.
Consider this: A marketing professional needs to curate images for a campaign. Instead of starting from scratch or relying on stock photos, they can use reverse image search to discover relevant visuals already in use in the market. In mere seconds, they can gather insight, inspiration, and connections, streamlining both the creative and decision-making processes.
By reducing the time spent on searches, this technology grants users a competitive edge, helping them focus on innovation and execution instead of data collection.
"Deep reverse image search is a game-changer. It streamlines workflows and boosts efficiency in an image-saturated world."
Limitations and Challenges
In the rapidly evolving landscape of technology, understanding the limitations and challenges of deep reverse image search is crucial. While this tool presents remarkable advancements, grasping its drawbacks can reveal potential pitfalls and inform better usage. Various factors play a role in how effective this search method can be, from the quality of image results to privacy and security concerns, as well as dependencies on database size and diversity. These elements deserve careful consideration as they directly impact user experience and the functionality of the technology.
Quality of Image Results
The quality of image results can greatly vary in a deep reverse image search. Contrary to the common expectation that the most precise images will resurface, users may find varying degrees of relevance. Factors such as image compression and scaling can distort input images, leading to mismatches.
Moreover, less refined algorithms may struggle with complex image details, failing to establish connections between visually similar objects. Some searches produce results that are akin to searching for a needle in a haystack, as the returned results may not exclusively match what one desires. This inconsistency can be particularly frustrating in critical sectors, such as law enforcement or medical fields, where accuracy is paramount.
One way to enhance result quality could be by integrating more advanced neural networks or refining the feature extraction techniques. In doing so, the efficacy of image retrieval can be improved without sacrificing the speed of searches.
Privacy Concerns and Data Security
With great power comes great responsibility, and the realm of deep reverse image search is no exception. Privacy concerns arise, particularly when users upload personal images for reverse searches. The digital footprint of these images can be traced, leading to unintended consequences if the data falls into the wrong hands.
Organizations often grapple with the task of safeguarding user data. Inadequate data protection measures could result in unauthorized access or leaks, exposing sensitive personal information. Furthermore, there is always the risk of misuse by individuals with malicious intent. Due to the potential for stalking or harassment, a deeper dialogue surrounding ethical boundaries in image use is essential.
To mitigate these risks, companies could implement stronger encryption methods and transparency measures, ensuring that users understand how their data is being utilized and protected. Being proactive about this can alleviate user concerns, fostering trust in the technology.
Dependence on Database Size and Diversity
The effectiveness of deep reverse image search is heavily dependent on the size and diversity of the image database it draws from. Imagine a library filled with books; if a particular subject is underrepresented, finding relevant information becomes a daunting task.
Similarly, if the database lacks variety, the search engine might struggle to return relevant results for niche queries. This limitation can hinder the search experience, especially for users seeking obscure visual content.
Moreover, a limited database can lead to biases in search results, reflecting only a narrow spectrum of images and potentially ignoring broader contexts. For enhanced performance, companies should prioritize expanding their databases while ensuring they encompass a wide array of content. By diversifying their datasets and continuously updating their collections, they can bolster the effectiveness and user satisfaction of deep reverse image search tools.
"Understanding these limitations helps us to harness the best of technology while remaining cautious of its pitfalls."
Ethical Considerations in Image Searching
In the vast realm of image searching, ethical considerations play a crucial role. As technology continues to evolve, the implications of using techniques like deep reverse image search extend beyond mere convenience. Grappling with these ethical dilemmas is essential for anyone engaging with this powerful tool.
Copyright Issues and Intellectual Property
When considering deep reverse image search, copyright issues emerge as a critical concern. The digital world is chock-full of images, many of which are protected by copyright laws. For example, when a user conducts a reverse image search to find the original source of a photo, they may inadvertently expose themselves to intellectual property violations.
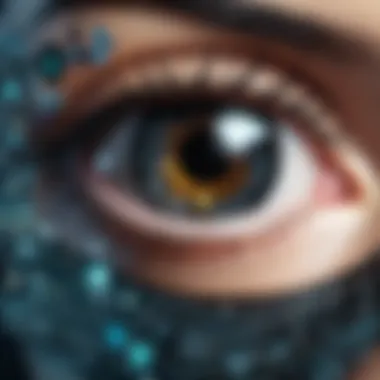
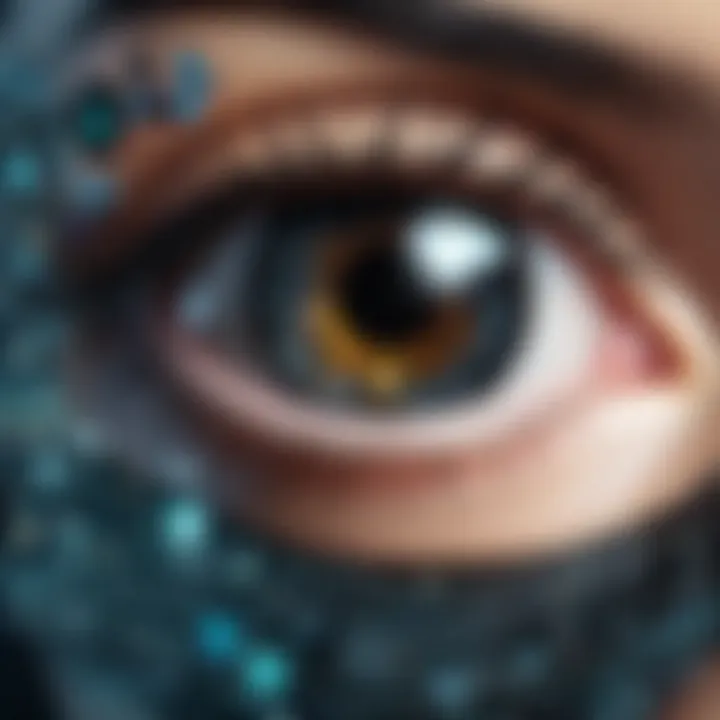
- Understanding Copyright: Copyright gives the creator exclusive rights to reproduce, distribute, and display their works. Without permission, using or sharing copyrighted images can lead to significant legal repercussions.
- Practical Impacts: Let's say a budding graphic designer comes across a stunning piece on social media and decides to use it in their project. If they fail to track down the original creator and obtain permission, they risk facing legal action.
- Navigating the Gray Area: Many users swim in murky waters surrounding fair use. Although fair use might apply in some cases, it is not a catch-all. Getting familiar with the nuances of copyright law is critical, especially when leveraging images found through reverse image searches.
Misuse and Malicious Intentions
Beyond copyright issues, the potential for misuse of deep reverse image search technology cannot be overstated. The ability to match images to their sources can easily be weaponized.
- Stalking and Privacy Invasion: One prominent risk is the capability to locate individuals. If someone uploads an image onto a platform and uses reverse image search, they may inadvertently expose their personal information. This can lead to unwanted attention from stalkers or harassers.
- Disinformation Campaigns: Images can be taken out of context, altering public perception. For instance, a viral photo from an unrelated event could be paired with a false narrative, spreading misinformation. Misuse of images in this way threatens the integrity of information in society.
- Artificial Intelligence Exploitation: Employing AI tools to generate fake images or manipulate existing ones is another concern. When users know how to leverage reverse image search, they might create and distribute malicious works, ultimately creating harm to reputations or personal lives.
"The line dividing what is acceptable and harmful in image searching is often blurry. Users must tread carefully to strike a balance between technology and ethical boundaries."
Navigating these ethical considerations demands vigilance and responsibility. Engaging deeply with ethical issues like copyright and potential misuse positions individuals not just as users of technology but as informed and conscious participants in the digital environment.
Future of Deep Reverse Image Search
The future of deep reverse image search holds immense potential, shaping the way we interact with images on the internet. This technology is not just about finding similar pictures; it's about transforming how businesses and individuals perceive and utilize visual information. With the continuous evolution of artificial intelligence and machine learning techniques, the importance of understanding the future of deep reverse image search cannot be overstated. It offers efficiency, accuracy, and expands the boundaries of creativity and innovation.
Technological Advances and Innovations
In recent times, a slew of technological advances has surfaced, making deep reverse image search far more sophisticated than just matching pixels. Neural networks are playing a crucial role in enhancing image recognition. These networks learn and adapt, significantly improving their ability to discern features within images. For instance, imagine an e-commerce platform struggling with users finding products. With deep learning algorithms, platforms can not only identify products in images but also suggest alternatives based on user preferences.
Another noteworthy advancement is the integration of augmented reality (AR) with reverse image searching. Users could point their camera at any object, and with a simple tap, related information pops up—be it identifying artwork, finding similar fashion items, or details about landmarks. This synergy between AR and image search transforms how we perceive the world while seeking information.
Furthermore, the incorporation of natural language processing (NLP) allows these image search engines to understand queries more contextually. Instead of mere descriptions like "red dress," one can input “find a similar party dress, that’s red and has a floral pattern.” With the advancement of multi-modal learning, these systems are poised to interpret both images and textual information simultaneously, bridging gaps in usability.
Potential for Industry Disruption
As we peer into the future, it's clear that deep reverse image search might disturb various industries in multifaceted ways. In the advertising sector, the ability to track where an image appears across the web can reshape brand visibility strategies. Companies will be able to gauge the effectiveness of their campaigns more accurately while ensuring their content is appearing where it should be.
Moreover, in the field of digital art and photography, creators can leverage these technologies to manage copyright better. Artists can track their work’s usage online, thus potentially reducing unauthorized use of their creations. Similarly, e-commerce giants are likely to adopt this technology to transform customer shopping experiences, eliminating the need for tedious text-based searches.
A few sectors that might see substantial changes include:
- Retail: Enhanced user experiences through visual search functionalities.
- Insurance: Quick claim processing via image verification technologies.
- Travel: Easy identification of locations through image queries.
"Innovation is not the product of logical thought, although the result is tied to logical structure." - Albert Einstein
As we move forward, the implications of deep reverse image search span beyond mere functionality; it beckons the dawn of new business models and operational paradigms. The outcomes of these advancements will likely raise new questions about ethics, privacy, and the very nature of content ownership in this rapidly changing digital landscape.
Finale and Final Thoughts
In the fast-evolving digital landscape, deep reverse image search stands out as a pivotal tool for both individuals and businesses. The technology not only streamlines the way we encounter and utilize visuals but also drives deeper engagements with media content. By harnessing advanced machine learning and artificial intelligence, these systems are capable of recognizing intricate patterns, allowing users to find similar images or even uncover the origins of specific ones. This capability can drastically transform how brands protect their images and how consumers interact with products online.
Summary of Key Insights
At its core, deep reverse image search leverages sophisticated algorithms to sift through vast amounts of data, pulling out relevant images based on specific visual features. The evolution of this technology highlights several key insights:
- Enhanced Accuracy: Deep learning methods enable a higher level of precision in retrieving image results.
- Broader Applications: From e-commerce to social media, the implications for various fields are profound.
- User Empowerment: It allows users to take charge of their digital footprints and better manage their online presence.
Despite its many advantages, a few fundamental aspects of deep reverse image search warrant consideration. Users should remain aware of potential drawbacks, like issues around privacy and database dependency. Balancing these concerns is crucial for both developers and end-users.
Implications for Users and Developers
The ultimate impact of deep reverse image search is twofold. For users, it enhances the ability to uncover visual content in new and innovative ways, allowing for richer interactions with media. The implications are substantial in sectors like online shopping, where consumers increasingly demand rapid access to information about products.
For developers, these advancements present both opportunities and challenges:
- Innovation Drive: There is a continued need to refine algorithms to enhance search capabilities.
- Ethical Design: Creating secure, user-friendly interfaces that respect privacy rights is paramount.
- Feedback Loop: Engaging with users to understand their needs can lead to continuous improvements in search functionality.
Through these dual lenses, the future of deep reverse image search seems promising. As technologies progress, we can expect deeper integrations into everyday applications, potentially influencing multiple domains from digital marketing to information retrieval. The road ahead will be shaped not only by technical advancements but also by the ethical framework surrounding the use of such powerful tools.
"Innovations in reverse image search technologies are not just about technology; they’re about understanding the implications for society and ensuring user rights are at the forefront."
The continuous evolution of deep reverse image search reminds us that the future holds vast potential. Embracing these changes while being mindful of their implications is essential for navigating this complex digital world.